Introduction
Are movies from Afghanistan associated with war? Was Paris always about love and romance? But most importantly... is Dracula present in every horror Romanian movie?
Welcome to "How does cinema view the world?", a data visualization journey through the cinematic world. In this project, we aim to answer these questions, uncover many other biases and analyse whether certain stereotypes are actually true.
The significance of the problem at hand is obvious, as these stereotypes can alter the way we perceive the world around us. Thus, our goal is to explore how cinema portrays the world, and its evolution over time. Biases can affect many aspects when it comes to movies. For example, some cities might be way more popular than others and have some specific genres associated with them in the cinema world. Perhaps, the location has an impact upon the characters and how they are represented. Or, it could be that we see drastic changes throughout time.
We will first give a general analysis for the entire cinematic world, countries and genres. Then, in the subsequent sections, we will explore in more detail cities, IMDb ratings, characters and timelines by clustering, plotting and many other techniques.
Understanding our data
Before we dive into the depths of our analysis, it's crucial to first examine our dataset closely. We utilized the CMU Movie Summary Corpus dataset, a comprehensive collection of 42,306 movie summaries. To enrich this dataset, we employed LLMs (OpenAI GPT-3.5-Turbo model) for generating the depicted locations, characters' alignments, and nationalities within the stories. A large part of our analysis is based on the information extracted using the LLMs, data that on its own is not always correct. However, we believe that the data is accurate enough to provide us with meaningful insights and to draw conclusions. We also employed a data cleaning process to remove any problems the data had.
Sample Data Representation: We have asked GPT-3.5 to give us a json formatted string detailing the cities, countries and characters in the plot. The model is prompted using the title of the movie and the summary.
Here's an example of an output from the model:
Movie Summary
The film begins with Moriarty and Holmes verbally sparring on the steps outside the Old Bailey where Moriarty has just been acquitted on a charge of murder due to lack of evidence. Holmes remarks, "You've a magnificent brain, Moriarty. I admire it. I admire it so much I'd like to present it, pickled in alcohol, to the London Medical Society". "It would make an impressive exhibit", replies Moriarty. Later Holmes and Watson are visited at 221B Baker Street by Ann Brandon. She tells him that her brother Lloyd Brandon has received a strange note - a drawing of a man with an albatross hanging around his neck - identical to one received by her father just before his brutal murder ten years before. Holmes deduces that the note is a warning and rushes to find Lloyd Brandon. However, he is too late, as Lloyd has been murdered by being strangled and having his skull crushed. Holmes investigates and attends a garden party, disguised as a music-hall entertainer, where he correctly believes an attempt will be made on Ann's life. Hearing her cries from a nearby park he captures her assailant, who turns out to be Gabriel Mateo, out for revenge on the Brandons for the murder of his father by Ann's father in a dispute over ownership of their South American mine. His murder weapon was a bolas. Mateo also reveals that it was Moriarty who urged him to seek revenge. Holmes realises that Moriarty is using the case as a distraction from his real crime, a crime that will stir the British Empire - an attempt to steal the Crown Jewels. Holmes rushes to the Tower of London to prevent the crime, and during a struggle Moriarty falls, presumably to his death. In the end, Ann is married and Holmes tries to shoo a fly by playing his violin, only to have Watson swat it with his newspaper remarking, "Elementary, my dear Holmes, elementary."
ChatGPT Output
{ "cities": [ "London" ], "countries": [ "UK" ], "characters": { "Sherlock Holmes": { "nationality": "UK", "alignment": "good" }, "Dr. Watson": { "nationality": "UK", "alignment": "good" }, "Moriarty": { "nationality": "UK", "alignment": "evil" }, "Ann Brandon": { "nationality": "UK", "alignment": "good" }, "Lloyd Brandon": { "nationality": "UK", "alignment": "good" }, "Gabriel Mateo": { "nationality": "South American", "alignment": "neutral" } } }
Using these outputs we have augmented our dataset and we can now start our analysis.
Large scale analysis
We will now show our first analysis section. We will start with a top-down approach, looking at the entire cinematic world, analyzing the countries and continents. We will delve into cities and characters in the subsequent sections.
After our preprocessing pipeline we have identified 21,000 movies with a country present and around 13,000 with a city present. Even with the lower numbers we still have a lot of data to work with and we can extract meaningful insights.
The distribution of plot locations across different countries is notably uneven. A majority of movies are set in the United States, followed by the United Kingdom and India. This imbalance is important to consider during analysis, as countries with a greater number of movies are likely to have more nuanced and diverse portrayals, potentially reducing stereotypes. The following is a visualization of the global distribution of plot locations.
Diving into Vector Space
With our analysis we wanted to uncover hard to find patterns that might not be easily representable in terms of genres as such we have decided to extract meaning from summaries in a less discrete fashion. We utilized embeddings, a technique to represent text as vectors. In this method, the meaning is encapsulated in the spatial distances between vectors. For our analysis, the OpenAI Ada2 model was employed to create these embeddings.
This approach allows us to quantify similarities between movies and to identify clusters. These clusters help us understand how movies are distributed across different countries and observe changes and patterns over time.
To provide a comprehensive view, we employed T-SNE for dimensionality reduction, making it easier to visualize these embeddings in two dimensions. Below, we can see the embedding space for all movies, with each point representing a movie. The colors represent the cosine similarity to the search term selected.
Through this visualization, we can discern meaningful clusters for most of the different keywords. For example, the Bollywood cluster is clearly visible, as is the War cluster.
We have also analyzed the clusters formed by countries in the embedding space. For instance, an analysis of German films reveals a cluster closely associated with war themes, whereas French cinema shows a significant cluster of love-themed movies, alongside war themes. The plot below allows switching between different countries and observing the clusters formed by movies from that country.
Interestingly, countries like the United States and the United Kingdom display a diverse range of themes, as opposed to countries like Vietnam, where most movies cluster around a singular theme. This could be due to the fact that the movie industry in the United States and the United Kingdom is more developed and has a larger variety of movies and are less influenced by the political and social events of the time.
Genre Analysis Across Countries
The CMU Movie Summary Corpus provides us with genres for each movie, allowing us to analyze the distribution of genres across different countries. As we are exploring biases in the movie industry, genre distributions come across as a very useful tool for comparing different locations.
We would expect a significant presence of war movies in Afghanistan, Vietnam, Germany; musicals in India; anime in Japan; martial arts and action movies in China; spy movies in Soviet Union.
On the contrary, few people would expect a significant number of comedy movies in North Korea, movies about business in Soviet Union, war films in Switzerland.
These prejudices are mostly dictated by cultural perception and historical events. In this section we want to focus on showing differences in genre distributions in different countries and proving that these differences are indeed statistically significant.
The plot below helps us to explore the most represented genres (at least 3% of all movies in the country) in different countries. Note that a lot of movies have more than one genre.
The plot confirms some stereotypes:
- Martial Arts and Action movies take place a lot in China.
- Japan, India and China are influenced a lot by the local movie industry (Japanese Movies, Bollywood and Chinese movies), on the contrary, European countries do not seem to have such a strong local movie genre presence, and rely more on worldwide genres like Drama, Comedy, etc.
- Germany, Vietnam and Afghanistan have a significant presence of war films.
- France lives up to its reputation in regards of Romance Movies.
We will later test these assumptions, to find statistically significant biases.
Let’s take a closer look at one notable genre: “War film”. Giving definitive locations for “Comedy” movies might be challenging, but for war movies a lot of places come to mind. We will take the top 10 countries where war films happen (filtered by countries with at least 100 movies, to filter out outliers).
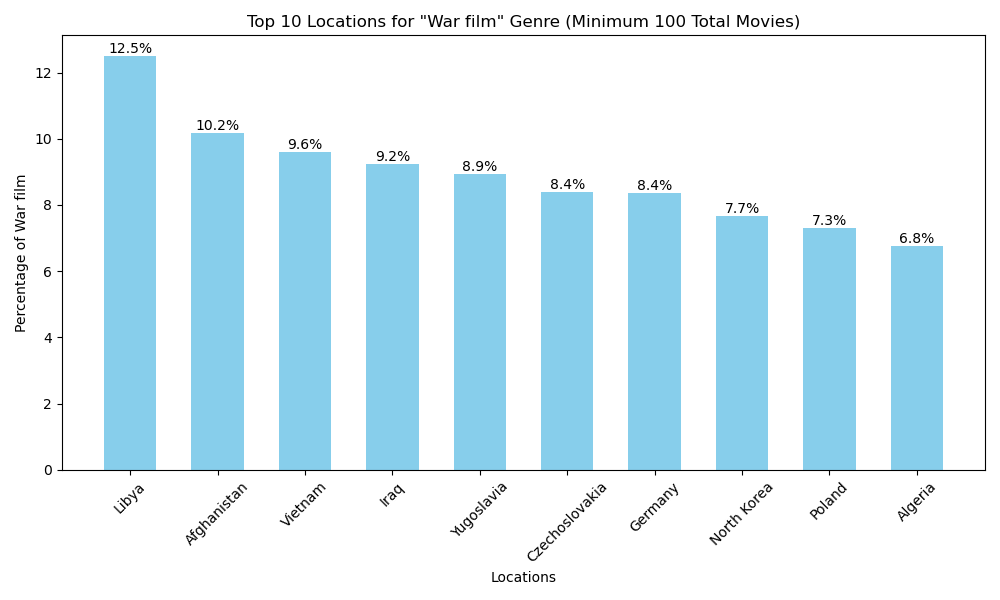
As seen in the plot, countries that are stereotypically associated with war can be found in the top 10. This means that we are on the right track, and should pursue statistical tests for different countries and genres to identify our biases.
Our analysis, backed by a Chi-Square test, reveals a significant disparity in movie genres based on the country. With a p-value close to 0, we confirm that movie genres are not uniformly distributed worldwide. This indicates a higher likelihood of certain genres being associated with specific countries.
Some notable examples confirmed include:
- France: Predominantly associated with romance movies.
- Afghanistan, Vietnam, and Germany: More inclined towards war-themed movies.
- India: Known for Romance and Musical movies, along with its signature Bollywood genre.
- Romania: Known for its Horror movies. Most notably, Dracula related movies.
We run proportions_ztest to identify if the proportion of genre in a country is significant. It also takes into account a general proportion of the genre, meaning that if a certain country has a lot of movies in total, but a small relative amount for the given genre, then it would not be considered significant, but if another country has a higher percentage of this genre, even given a lower number of movies for the genre compared to the first country, this will be marked as significant. We run this test on some stereotypical country-genre pairs to prove the statistical significance. We include some unexpected location-genre pairs (e.g. North Korea - Comedy) to perform a sanity check on our test.
As seen in the table, our hypotheses were proven with a significance test. Indeed, Germany has a significant proportion of war and biographical movies; France has a lot of romance and art films and not significantly many musicals; on the contrary, India has a very significant presence of musicals; Soviet Union lives up to its reputation with many Spy movies, and expectedly is not associated with Comedy (these both are also the case for North Korea); Japan has significant presence of anime, but China doesn’t; however, China has indeed a lot of martial arts movies; Afghanistan is notable for war films; Romania has a significant amount of horror movies.
As we’ve discovered some individual cases of significance testing, we will run significance tests for all possible country-genre pairs and visualize how certain countries have significant presence of certain genres. As we are running many tests simultaneously, we are using Bonferroni correction on our p-value threshold due to an increased risk of a “type I” error when making multiple statistical tests.
This table captures statistically significant country-genre relations. The observations serve as a strong argument of bias in movies.
IMDb Ratings Analysis
This subsection delves into the analysis of IMDb ratings, focusing on variations across different countries and continents. Our findings indicate notable differences in movie ratings based on the location and shows positive and negative biases in the film industry. The plot below shows the average IMDb rating for each continent accross a number of genres. We can already observe some interesting patterns.
In order to formally analyze our data we have utilized an ANOVA test which revealed significant variances in the IMDb ratings based on the continent. To further explore these differences, we conducted a Tukey test to pinpoint continents with notably distinct ratings.
Key insights from the Tukey test include:
- South America: Generally exhibits lower average ratings across multiple genres compared to other continents.
- Europe: Stands out with significantly higher average ratings in romance movies.
Cityscapes
Cities are a central part of many movies. They are often used as a backdrop for the story and can even be a character in their own right. We will look at how cities are portrayed in movies and what biases are present.
Most Popular Cities
Let's first compute the most popular cities (where the movie plot takes places) for our Top 10 most represented countries. We will show the proportion of movies that were produced by the country in which the city is located, as opposed to those that were made by foreign countries.
Looking at these 10 countries, we often see that the most popular city in movies is also one of the biggest, most populated cities in the country.
Mumbai stands out with a high number of local films, showing India's strong film industry or the little interest of foreign countries.
On the other hand, Rome, with its rich history, seems to be a favorite for filmmakers from around the world, having the highest foreign representation among these cities.
Hong Kong is really interesting because even though it's not in the top 10 of the biggest cities in China, it's highly represented in movies, probably due to its history
of being a colony of the British Empire before transfering to China in 1997.
Let's now dive further and looking into which of these foreign countries make the most movie plots talking place in a particular city.
We will look into Berlin, which has a high percentage of 66% of foreign produced movies.
As we might have predicted, the United States is a major contributor to the movie industry and has produced more movies set in Berlin than any other country. This considerable impact may lead to a certain bias in the cinematic image of Berlin as it has primarily been depicted by American filmmakers.
Top 10 Cities
We can now showcase the ten most appreciated cities for movie narratives in general and see if there are any trends or anomalies. These cities are likely the ones that viewers have the most preconceived notions about, even if they have never visited them in their lives.
Five of these cities are in the United States, collectively representing 25.6% of movie plot locations. This is unsurprising, given that the majority of the world's movies are produced in the United States. Nevertheless, this significant influence can be seen as a form of soft power, potentially shaping foreign viewers' perceptions of these cities.
Genre Distributions Across Cities
As we’ve explored genre biases in countries, we now zoom in to look at cities. While one might expect the genre distributions in Paris and Tokyo to align with those of France and Japan, respectively, the situations in New York and Las Vegas could present more variation, both from each other and from the overall genre distribution of the United States.
IMDb ratings
Is it better to make a movie about New York or London? Let's capture IMDb ratings based on cities in the movies.
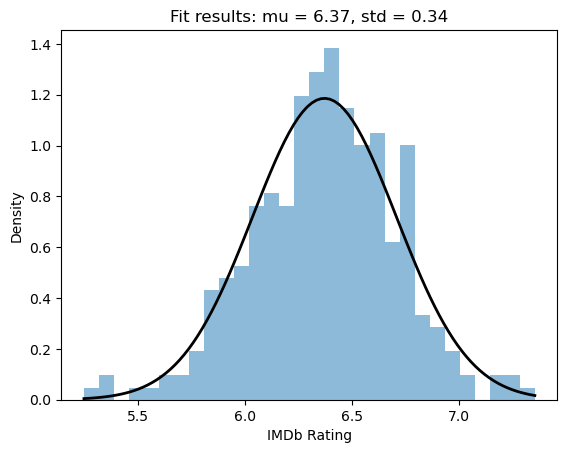
The Gaussian distribution of IMDb ratings gives us an initial indication that there is no significant difference in the average ratings of movies between countries, however, we will delve deeper to check this. Given this, we zoom in to visualize IMDb rating differences in four informative genres: Romance, Martial Art, Bollywood, Horror.
Key Observations:
- Romance: Paris performs really well in this genre. Being similar to London by the number of romance movies and votes, it gets 6.66 against 6.38 in London.
- Horror: Movies about Transylvania are ranking the highest, given a fame of the region.
- Martial Arts: Hong Kong is a clear winner with a biggest amount of Martial Arts movies and a rating of 6.34
- Bollywood: This genre is dominated by Indian cities, with an exception of London, which, performs the worst out of major cities, and New York, which is almost on par with Delhi and Mumbai. Very high rating in Calcutta might be explained by a small number of movies associated with it (17).
We can see that the distribution of IMDb ratings is not uniform across cities. We have performed the Tukey test to see which cities have significantly different ratings.
Characters
Characters are the heart of any story. They are the ones that drive the plot forward and make us care about the story. We will look at how characters are portrayed in movies and what biases are present.
Character alignments by countries
The following graphs provide insights into the alignment of characters in movies per countries, with each time varying the threshold representing the minimum of characters per country. In fact, we defined a minimum number of characters that a country must have for its data to be included in our analysis. This threshold is essential to ensure that the data for each country is sufficiently representative. It would not be fair or accurate to draw conclusions from a country's data if it's based on, say, just one evil character.
For instance, we found that a threshold of 10 characters is great to establish a representative sample and including a diverse range of countries in the analysis. This number is substantial enough to mitigate the risk of skewing the results with outliers (such as a single character portraying a negative stereotype), yet not so high that it excludes countries with lesser representation in global cinema. Countries that don't meet this minimum threshold are coloured in grey on the world map. This way, we can include countries that have a moderate presence in films while ensuring that their portrayal isn't dominated by a few characters.
Ratio of evil(0) to good(1) characters by country
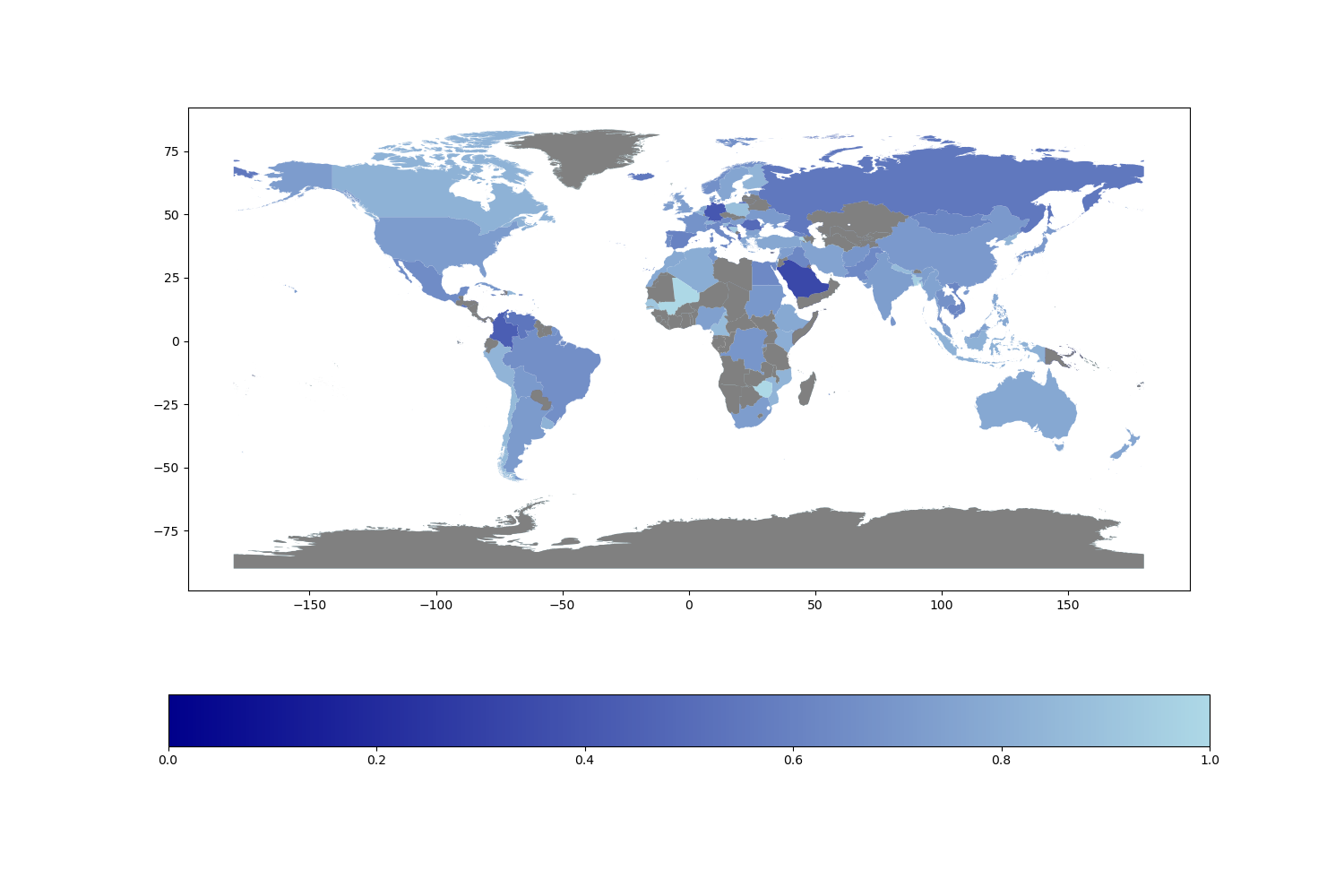
The countries in lighter blue have a higher proportion of good characters, while those in darker blue have a lower proportion. This distinction helps us quickly assess the global distribution of character alignments and understand potential biases in character representation based on nationality in the film industry.
Additionally, selecting a fairly high minimum number of characters, like 400 or 800 as shown in the graphs, allows us to identify the most represented countries in global cinema and those that are not. This higher threshold highlights the countries with a significant presence in films, offering a clearer picture of character distribution on a larger scale. Here is a table regrouping the top 5 countries regarding the number of characters.
COUNTRY | NUMBER OF CHARACTERS |
---|---|
United States of America | 29'002 |
India | 9'552 |
United Kingdom | 2'959 |
Japan | 2'044 |
France | 1'778 |
The following table reveals: the top 7 countries with the lowest evil to good ratio, where characters tend to have more evil alignments, and the top 7 countries with the highest ratios, indicating a prevalence of good character alignments. This comparison offers a unique perspective on how different countries are represented in terms of character morality in global cinema.
COUNTRY | RATIO | GOOD CHARACTERS | EVIL CHARACTERS | TOTAL |
---|---|---|---|---|
Saudi Arabia | 0.33 | 8 | 16 | 24 |
Germany | 0.40 | 558 | 828 | 1'386 |
Colombia | 0.43 | 36 | 47 | 83 |
Romania | 0.49 | 66 | 69 | 135 |
Albania | 0.52 | 11 | 10 | 21 |
Serbia | 0.53 | 46 | 40 | 86 |
Venezuela | 0.55 | 18 | 15 | 33 |
... Countries in the middle ... | ||||
Poland | 0.88 | 141 | 19 | 160 |
Senegal | 0.92 | 33 | 3 | 36 |
Bosnia and Herzegovina | 0.93 | 13 | 1 | 14 |
Bangladesh | 0.95 | 36 | 2 | 38 |
Mali | 1.00 | 10 | 0 | 10 |
Armenia | 1.00 | 15 | 0 | 15 |
Zimbabwe | 1.00 | 11 | 0 | 11 |
This table, illustrating the moral alignment of characters from various countries, uncovers intriguing patterns in global cinema. Countries like Saudi Arabia and Germany predominantly portray evil characters, while others like Bangladesh and Armenia lean towards good characters. For Colombia for instance, one might speculate that its history and the global spotlight on drug trafficking themes may play a role in this depiction. Such disparities raise questions about cultural stereotypes and biases in film. Notably, the under representation of African nations, evident in many greyed-out countries on the map, highlights a significant gap in the cinematic narrative.
Character names by countries
Moving beyond character alignments, we look into the character names across different countries. These word clouds visually capture the essence of naming conventions in the global film industry, highlighting the most frequently occurring names within each nation.
Word cloud per country
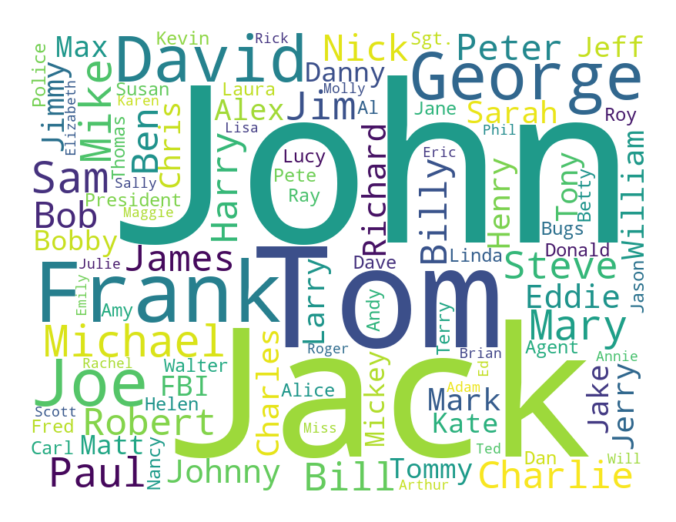
This visualization displays the word clouds, where the size of each name represents its frequency or prevalence in the dataset. These word clouds provide a graphical representation of the most common names associated with characters from different national backgrounds in movies, offering an interesting cultural insight into name usage in cinematic contexts. For instance, we see "Jan" as a prominent name in the Netherlands word cloud, reflecting its commonality in Dutch culture. Similarly, classic Western names dominate the clouds for the United States and France, and the Indian cloud shows a mix of traditional Indian names.
Regarding the German word cloud, the presence of "Adolf" and "Nazi" shows how strongly certain historical narratives persist in cinema. These words being prominent suggest a significant number of films focus on World War II themes, which may overshadow other aspects of German culture and history in cinematic storytelling.
Going back in time
Movies are a reflection of the time they were made in. They are influenced by the culture and events of the time. We will look at how movies have changed over time and what biases are present. One of the first observations made is that the number of movies increases over time, as we can see in the plot below.
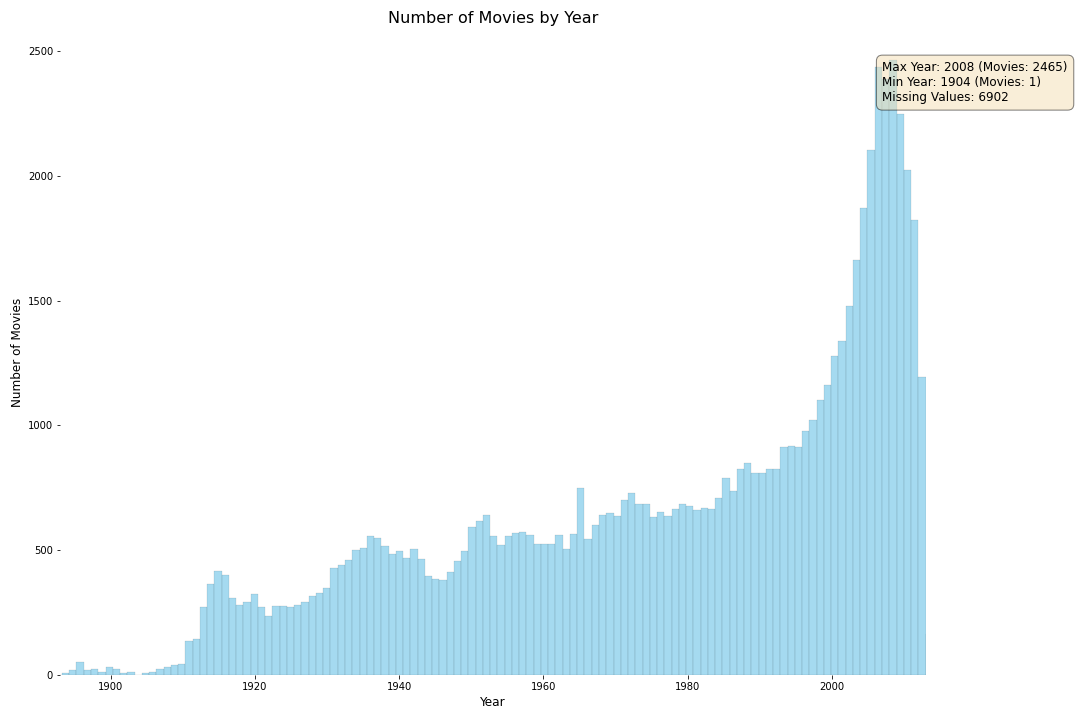
The clear growth depicted in the movie industry can be attributed to a combination of factors. Technological advancements over the last few decades have played a significant role, making filming equipment more accessible and affordable. This has empowered filmmakers worldwide, allowing for a surge in creative expression. Additionally, the phenomenon can be linked to the broader trend of globalization. Films from diverse cultures and countries have garnered increased international recognition, contributing to the overall expansion of the industry. Notably, the interplay between globalization and various social and political changes has likely influenced a notable shift in movie genres. As narratives and storytelling reflect the evolving global landscape, audiences witness the emergence of new and diverse cinematic themes.
In order to investigate this aspect, we can analyze the embedding space below.
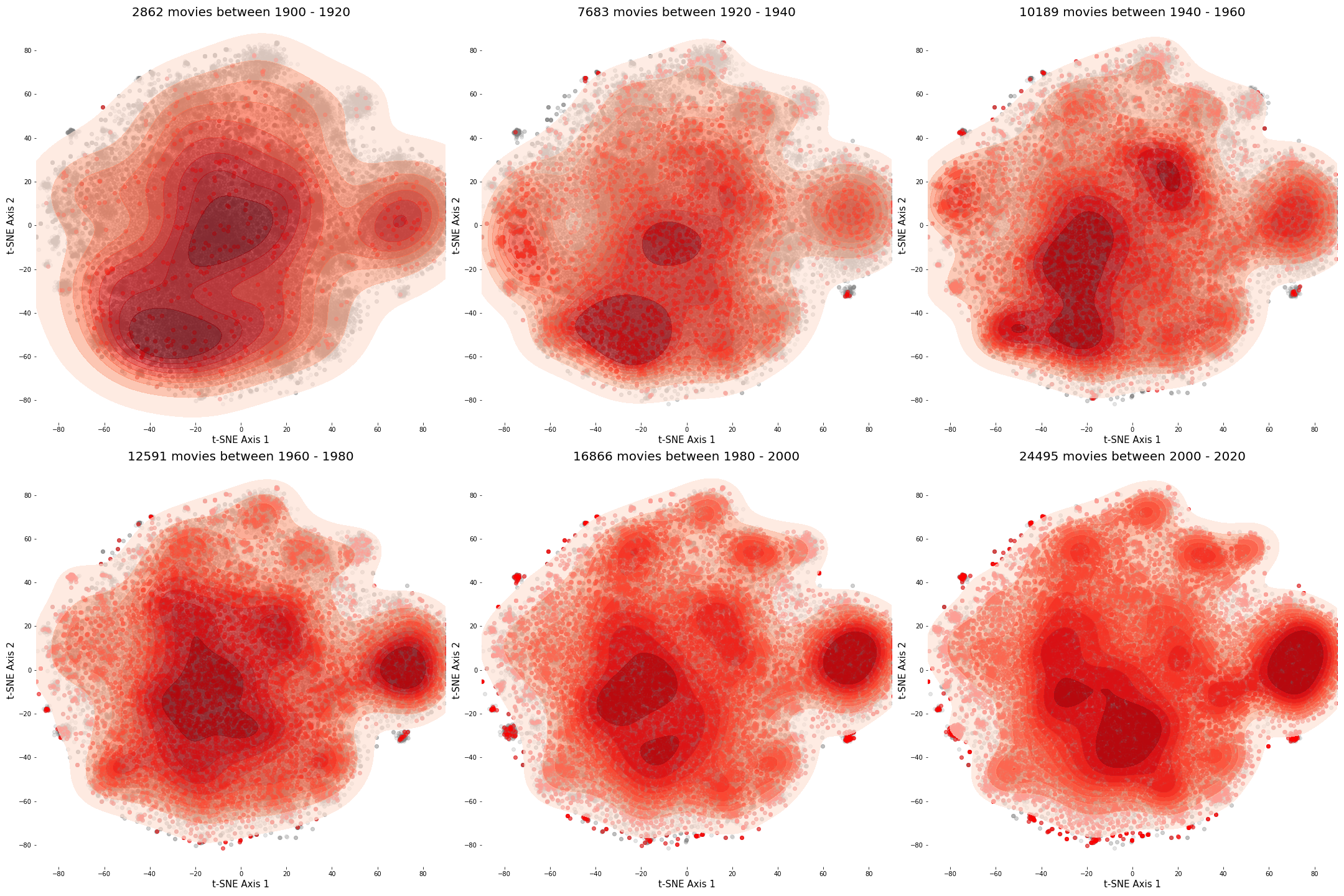
The changes observed in this embedding space align with significant political and social events throughout the 20th century. Moments such as World War II, the Cold War, and other very important historical occurrences have left a clear mark globally. It is within these crucial periods that we observe the division and formation of distinct clusters in the embedding space. The dynamics of these clusters likely mirror the shifts in societal perspectives, cultural preferences, and thematic choices within the film industry. This correlation suggests that the embedding space serves as a nuanced reflection of historical and societal changes, providing valuable insights into the interplay between cinema and the broader world around it. Thus, the exploration of these patterns can deepen our understanding of how such global events and cultural shifts are depicted in the cinematic world.
Genre evolution
In this section we aim to visualize how genre distributions in different countries were evolving due to historical events and trends.
- World War II is one such event, the effect is clearly seen with a rapid growth in the plots of France, Germany and the United Kingdom.
- Another trend is the rise of Martial Arts and Action movies in Hong Kong during the 1970s.
During the 1970s and 1980s, the most visible presence of martial arts films was the hundreds of English-dubbed kung fu and ninja films produced by the Shaw Brothers, Godfrey Ho, and other Hong Kong producers. These films were widely broadcast on North American television on weekend timeslots that were often colloquially known as Kung Fu Theater, Black Belt Theater, or variations thereof. Included in this list of films are commercial classics like The Big Boss, Drunken Master, and One Armed Boxer. Martial arts films have been produced all over the world, but the genre has been dominated by Hong Kong action cinema, peaking from 1971 with the rise of Bruce Lee until the mid-1990s with a general decline in the industry, until it was revived close to the 2000s. Wikipedia
- Decline of British Empire films and rise of Bollywood and Musicals in India after gaining independence in 1947.
- Vanishing of Black-and-white movies in France (there were so many movies, that it still remains a major genre in France, 3.68%, 237 movies)
Cluster time view by country
Looking at each country in particular we can observe some interesting patterns.
We can see that the embedding space for each country is different across the time intervals. We can observe that the war cluster is present in the 1940-1960 interval for all countries but some countries have a more pronounced war cluster than others. For example, Germany has a very pronounced war cluster, while the United States has a more diverse embedding space. The German cluster does not seem to go away in the subsequent time intervals, while for example France losses it quite quickly.
Case study: France
A country which is very interesting to analyze, due to its major changes in movie genres is France. Firstly, we depict two very important clusters for our analysis: the war and the love clusters.
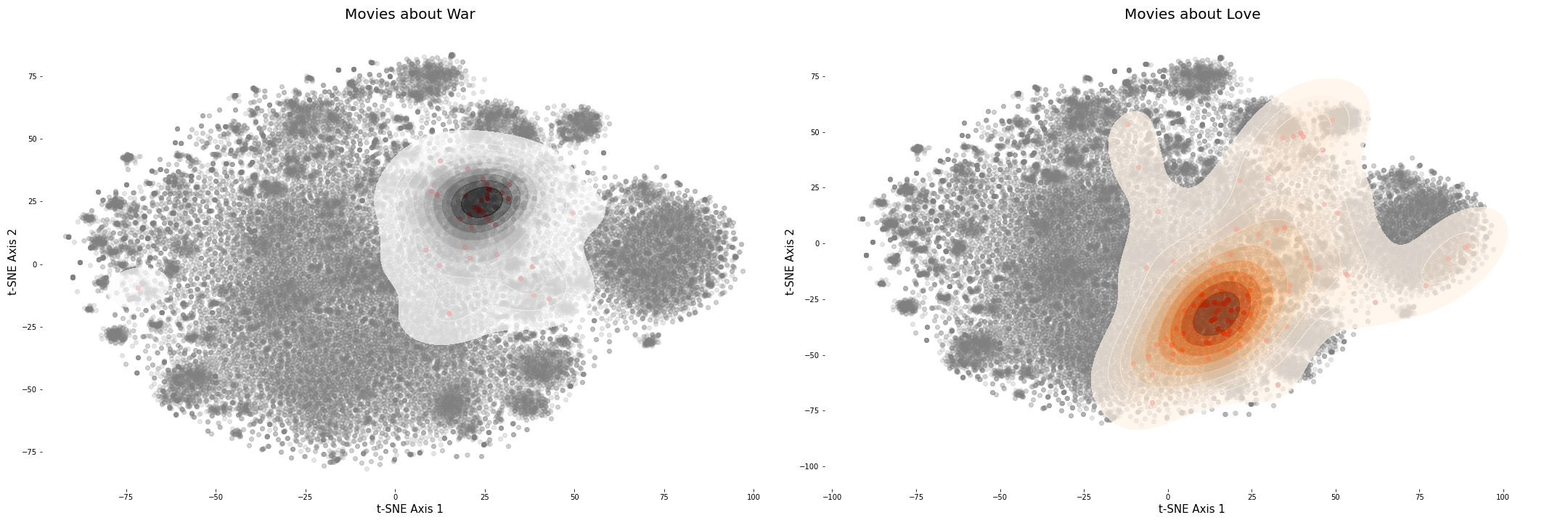
Both are positioned on the right side of the embedding space, with one positioned in the lower section and the other in the upper section. It turns out that these two clusters are crucial when it comes to analyzing France, as we notice a slow transition from one to the other. Below, we can visualize the plot with the movies in France, from 1900 to 2020.
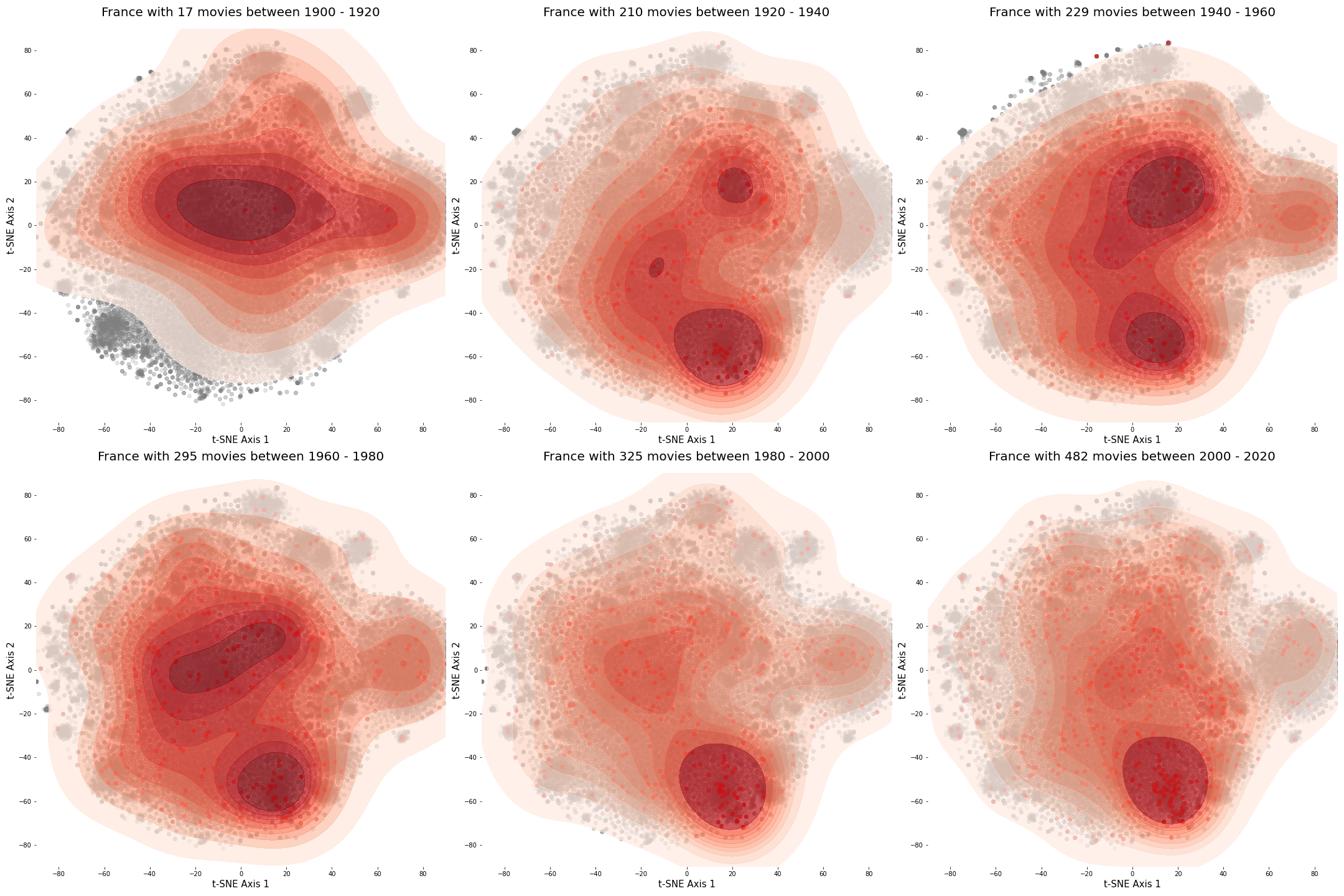
In the plot depicting movies from 1940 to 1960, we see many films present in the war cluster. However, as we move through the subsequent periods—1960 to 1980, 1980 to 2000, and 2000 to 2020 — we observe a notable absence of movies associated with the war cluster. Instead, there is a gradual transition to romance genres, reflecting historical events. As time progresses from World War II, its significance diminishes, and movies return to the roots.
Conclusion
In conclusion, our journey through the world of cinema was a challenge in continually shifting perspectives to identify and question underlying stereotypes and biases. By looking into various aspects such as genre distribution across countries, the portrayal of cities, character alignments and names, and the evolution of cinematic themes over time, we have uncovered a variety of narratives that reflect both global diversity and specific cultural biases.
Our findings highlight the significant role cinema plays in shaping perceptions and reinforcing stereotypes. From the dominance of certain genres in specific countries to the evolution of themes over decades, films often mirror societal values, historical events, and cultural shifts. The portrayal of cities and the alignment of characters in different countries also contribute to a nuanced understanding of global storytelling practices.
This project underscores the importance of viewing cinema not just as entertainment, but also as a lens through which we can understand the world. The biases and trends we identified prompt a more critical and informed approach to consuming film. As we continue to engage with cinema, let it be with an awareness of its power to both reflect and shape our understanding of diverse cultures and histories.